Unraveling Bias: Confronting Fairness Challenges in AI Systems
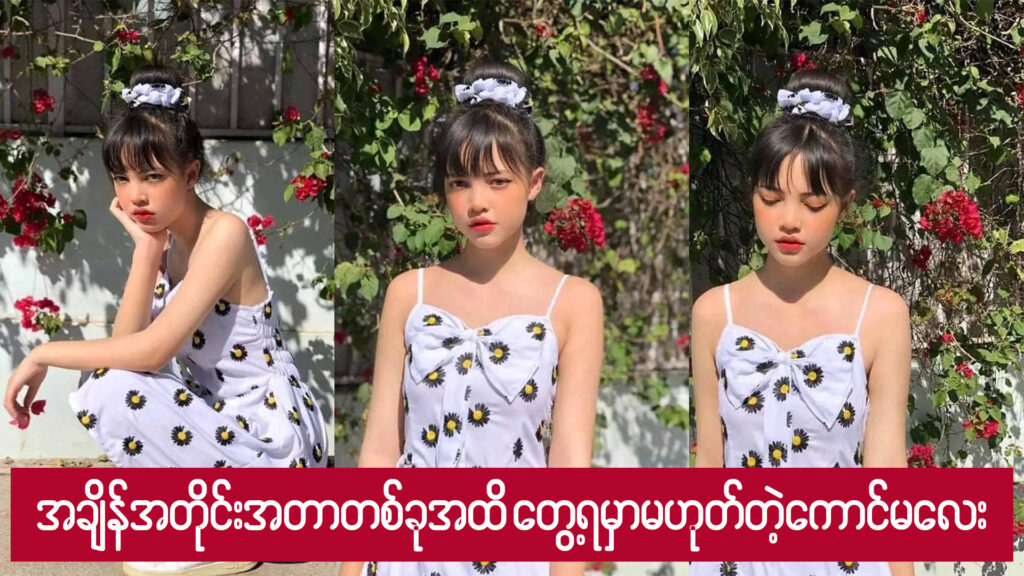

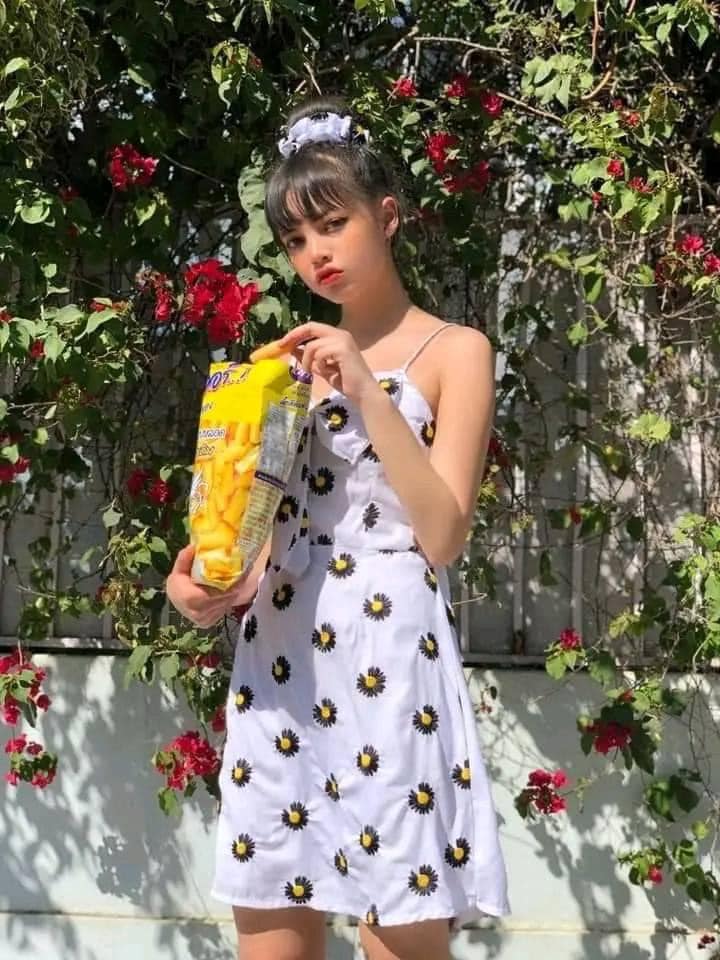
In the realm of artificial intelligence (AI), the pursuit of fairness has become paramount. As AI systems increasingly shape various aspects of our lives, from hiring decisions to criminal justice sentencing, the presence of bias within these systems poses significant challenges. Addressing bias in AI is not merely an ethical imperative but also a practical necessity to ensure equitable outcomes for all individuals.
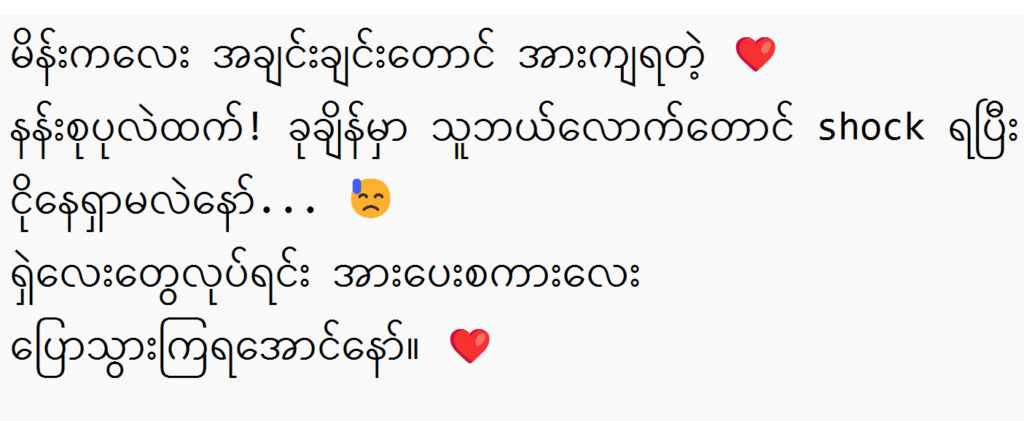
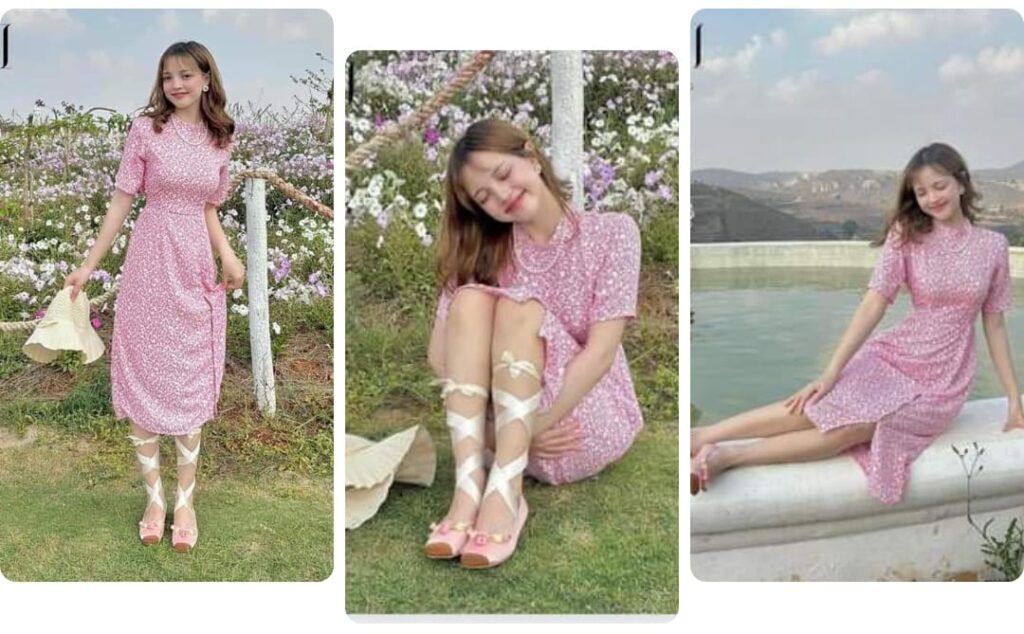
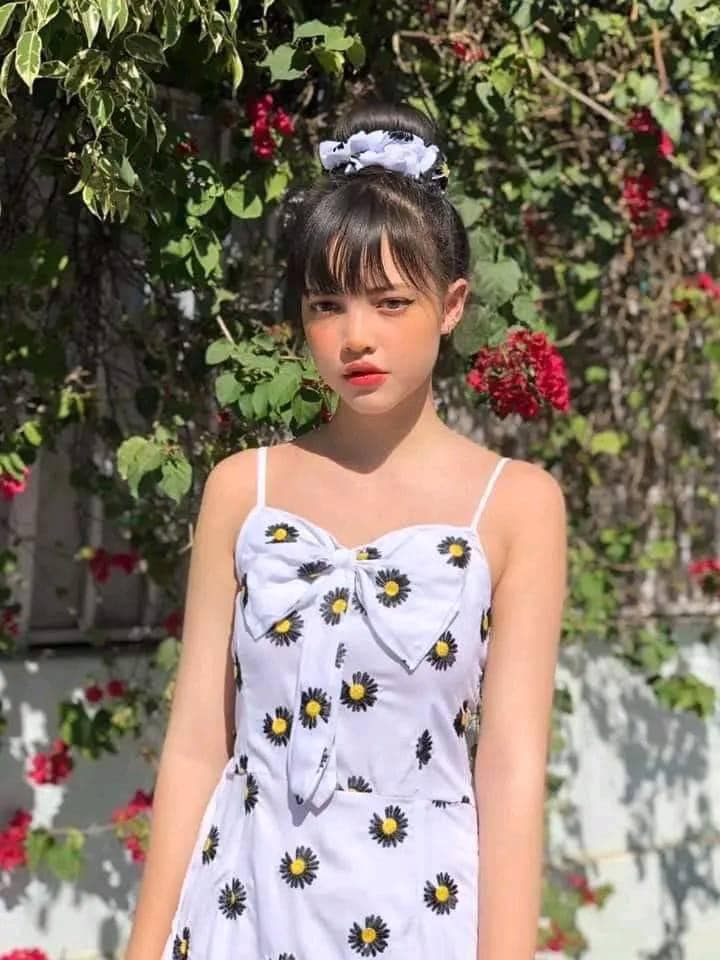
Bias in AI systems often stems from the data used to train them. Historical data reflecting societal biases and prejudices can perpetuate and even exacerbate existing inequalities when fed into AI algorithms. For example, biased hiring practices may be inadvertently encoded into AI-powered recruitment tools, leading to the perpetuation of gender, racial, or socioeconomic disparities in employment opportunities.
Furthermore, the algorithms themselves may introduce or amplify biases through their design and optimization processes. If AI systems are trained on data that primarily represents certain demographic groups or perspectives, they may struggle to accurately generalize to other populations, resulting in unfair outcomes for marginalized communities.
Addressing bias in AI requires a multifaceted approach that involves both technical and ethical considerations. One crucial step is to ensure diversity and inclusivity in the data used to train AI systems. By incorporating diverse datasets that accurately represent the full spectrum of human experiences, developers can mitigate the risk of perpetuating biases in their algorithms.
Additionally, transparency and accountability are essential pillars in the quest for fairness in AI. Developers must be transparent about the data sources, methodologies, and decision-making processes underlying their AI systems. This transparency allows stakeholders to assess the potential biases present in AI algorithms and hold developers accountable for any harmful outcomes.
Moreover, ongoing monitoring and evaluation are necessary to detect and mitigate bias in AI systems post-deployment. Regular audits and assessments can help identify instances of unfairness or unintended consequences and prompt adjustments to the algorithms or decision-making processes accordingly.
Ethical considerations also play a vital role in ensuring fairness in AI systems. Developers must prioritize ethical principles such as fairness, transparency, and accountability throughout the AI development lifecycle. By embedding these principles into the design, deployment, and governance of AI systems, developers can help mitigate the risk of bias and promote equitable outcomes.
Furthermore, promoting diversity and inclusivity within the AI development community is crucial for addressing bias. By fostering diverse perspectives and experiences among AI researchers and practitioners, the field can better identify and mitigate biases that may otherwise go unnoticed.
In conclusion, addressing bias in AI systems is a complex and multifaceted challenge that requires concerted efforts from all stakeholders. By prioritizing diversity, transparency, accountability, and ethical considerations, developers can work towards building AI systems that promote fairness and equity for all individuals. As AI continues to shape our world, it is imperative that we break down bias and strive towards creating a more just and inclusive future.